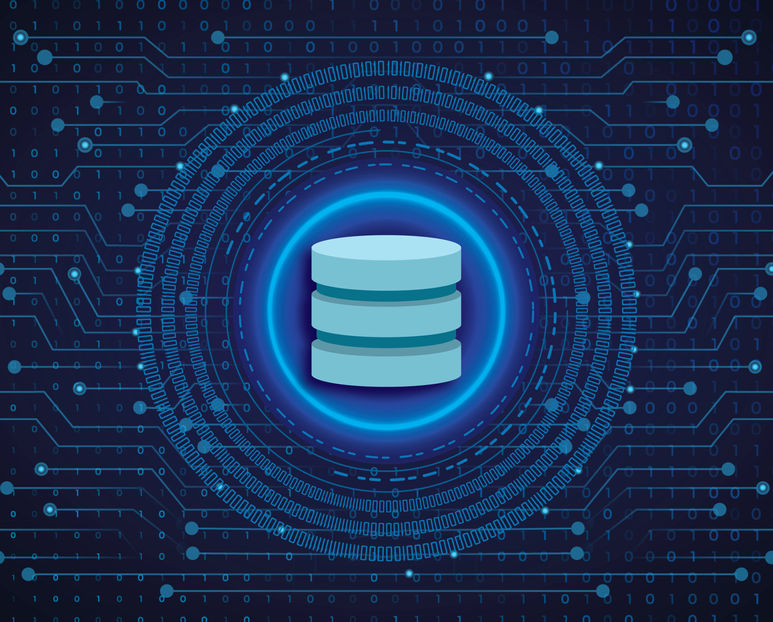
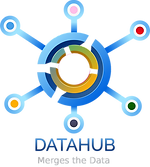
DataHUB
In the digital world, Data is everything. Businesses rely on data to make decisions, understand trends and evaluate performance. As data becomes more and more central to how businesses operate, data quality becomes more important. Data quality refers to the accuracy, completeness, timeliness and consistency of data. In other words, it's about making sure the data is clean, accurate, and up-to-date. If the data is unreliable, any insight or decision based on that data will also be unreliable.
Poor data quality can negatively impact business performance, leading to inaccurate analysis and poor decision making. That's why data quality is so important - essential for making sound business decisions. Data quality is one of the key components of data governance. Data governance is a framework for managing data throughout its lifecycle. It includes processes and procedures for receiving, storing, using and destroying data.
The main features sought in data quality are as follows;
It can be defined as the degree to which data meets user requirements. For example, if you are measuring the sales of a product, the data should accurately reflect how much of that product has been sold. Some points to be considered in data accuracy;
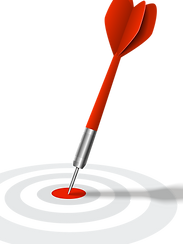
Data Accuracy
Incorrect data: It is data that does not reflect reality. For example, if expenditure data is incorrectly associated with a different expenditure category, it will result in an inaccurate analysis.
Duplicate data: Records that appear more than once in the dataset. For example, if you have customer records with two different records for the same customer, this is considered duplicate data.
Missing data: Data that should be present in the dataset but are not.
Up-to-dateness can be defined as the degree to which data reflects the current state. For example, if you are measuring the number of products sold, last year's data will not be considered current data.
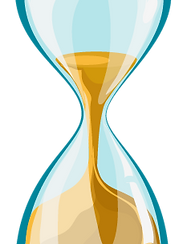
Data Timeliness
Old data: Data that is no longer accurate because it reflects a past time period. For example, last month's data is considered old data.
It can be defined as the degree to which all desired information is found in a data set. In data integrity, the answers to these five questions are generally sought; Who, what, when, where and why.
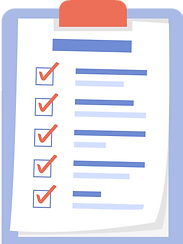
Data Copleteness
Missing data: It is data that is missing information. For example, if you only have partial customer data, it is considered incomplete data.
Irrelevant data:Data that is not relevant to the question asked. For example, if you are measuring the number of products sold, data on the number of employees in the company is considered irrelevant data.
Data consistency can be defined as the degree to which data is the same across different datasets. For example, if you have customer data from two different sources and the data is inconsistent (for example, one source has an email address and the other does not), then it is considered inconsistent data.
Data Integrity
Data integrity can be defined as the degree to which data has not been altered from its original state. For example, if you have customer data and someone has changed the data (eg, changed their email address), this is considered a data integrity issue.
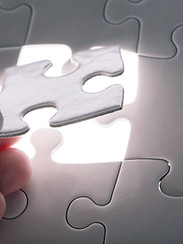
Data Consistency
Different data formats: Data that is formatted differently from the desired format. For example, if you have customer data in a text file, but you want it in a CSV file, that data is treated in a different format.
Structured and unstructured data: Data that is organized in a particular way (for example, in a table) or not organized at all. For example, text data is considered unstructured data, while data in a CSV file is considered structured data.
Example One
DataHUB Model
